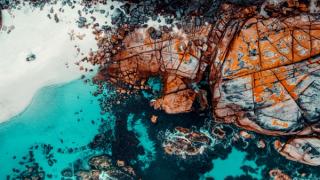
Climate Transition Plans: Decarbonisation is only one part of the puzzle
by James Balik-Meacher
View post
Camera traps provide a cost effective and unobtrusive way to monitor and survey animals in their natural habitats. Camera trap technology has continued to advance to meet the demands of modern-day scientists.
What were once bulky, complex pieces of equipment with minimal battery life and low-quality image outputs have now become sleek, lightweight units that can capture sharp, high-resolution images over vast periods of time, giving scientists an insight into the private life of animals.
However, with continued advancements in battery longevity and camera quality, photo files collected from camera traps continue to grow in size and number. The results leave scientists with expansive digital data sets, some of which can take multiple months to sort, catalogue, and analyze.
The large data outputs pose one of the biggest challenges with this style of data collection. Another challenge that scientists face when using camera trap technology is the inability to frequently recognize individual animals, which don’t have unique markings or identifiable features.
When animals can be individually identified (e.g., colored ear-tags on large mammals, or unique natural markings on the body like scratches on whales) a camera trap data set has a number of positive implications for ecological research and conservation of the species being studied.
These implications include estimations of population size, habitat range and movements of individual animals, as well as, assisting with human/wildlife conflict through the identification and management of problem animals.
Scientists have recently begun using artificial intelligence (AI), specifically machine learning (ML), to assist with data classification and animal identification challenges. Although this technology is relatively new and poses its own set of challenges, the practical applications and future implications are hard to ignore.
A number of research biologists and software specialists across the globe are working to incorporate AI classification and identification into a broad range of scientific projects.
The BearID Project, made up of research biologists from the University of Victoria and a small software development team, is using deep learning to identify individual brown bears from camera trap data sets. Deep learning is a subset of ML, which uses large neural networks to learn data representations from training data.
Deep learning is often used for human facial recognition, and commonly yields more than 99% mean classification accuracy. The BearID project is developing software that utilizes the same techniques used for human facial recognition and adapting them for the identification of individual bears, automatically scanning images and videos from camera traps to find and identify any bears present in the data set.
Brown bears caught by camera traps are not always easily identified, due to a lack of unique natural markings. However, researchers from the BearID project are hopeful that this new technology will change that.
In addition to addressing the current inability to identify some brown bears, researchers at the BearID Project are hopeful that this technology will have a variety of applications such as resolving human/wildlife conflict with the better management of problem bears, diversifying the type of data recorded, speeding up analysis of camera trap data, and allowing expansive data sets to be collected and analyzed efficiently.
The BearID software has shown an impressive initial classification accuracy rate of 84%. Researchers are hopeful that they can improve on this with the addition of a larger data set of photos for the AI to learn from.
Photograph of a Bear taken by author Kristian Gillies.
Deep learning has also proved to be an effective means of automating animal identification on large scale data sets.
A collection of researchers from the University of Wyoming, Auburn University, Harvard University, University of Oxford, and the University of Minnesota were able to train convolutional neural networks (a class of deep learning) to identify, count and describe behaviors of 48 species across a 3.2 million image data set taken from the Snapshot Serengeti (SS) camera trap dataset.
The SS project is the world’s largest camera trap project to date, collecting images on 225 remote cameras across a wide variety of habitat features in Serengeti National Park, Tanzania. The SS project used over 19,000 volunteers to assist with the classification and analysis of the large camera trap data set, enabling these researchers to compare the accuracy and efficiency of deep learning AI technology to human effort.
The team discovered that if their deep learning system classified only images it was confident in, it was able to automate animal identification for 99.3% of the 3.2 million image data set while performing at the same 99.6% accuracy rate of the crowdsourced volunteers - saving more than 8 years of human effort (17,000 hours at 40 hours/week). This study provides a strong argument for the use of AI technology to assist with the classification and analysis of large-scale camera trap data sets.
One of the more interesting applications of AI technology is Wildlife Insights, an AI-enabled feature on Google Cloud Platform.
Wildlife Insights provides a cloud service for scientists to upload large camera trap data sets and run Google’s species identification models over them. The creators have trained an AI model to automatically classify species within an image, using Google’s open sourced TensorFlow framework.
Google’s AI models have been trained on a dataset of 11.6 million photos (the largest curated dataset of labeled camera trap images to date) and can identify 732 different species with an 80 to 98.6% probability of correct prediction.
The second function of Wildlife Insights is the ability to automatically remove images that do not contain animals from within a data set, known as blank image filtering. The AI models used for blank image filtering can catch 47% of blank images with an error rate of 2.5%, essentially halving the time required to filter out blank images from camera trap data sets.
Wildlife Insights not only provides a prospective system to identify animals and filter blank images from large scale data sets, but it is also creating a platform for both researchers and citizen scientists alike to collaborate and share data, forming the framework of a global monitoring network.
The continual evolution of camera trap technology will lead to larger datasets, containing higher resolution imagery, captured over longer periods of time.
This will advance our knowledge on long term trends as well as expose minute details in the data, which may have previously been overlooked; henceforth allowing for a greater understanding of the best management practices for ecosystems and the animals within them.
At this point in time, it is difficult to place a price tag or time stamp on this technology and when it will become readily available for private consultants to use; however, as datasets and image quality grow, so will the accuracy and efficacy of deep learning AI, a technology that is likely to become an essential counterpart to camera trap data analysis in years to come.
by James Balik-Meacher
by Peter Lo
by Melissa Birch, Tarra Tamang